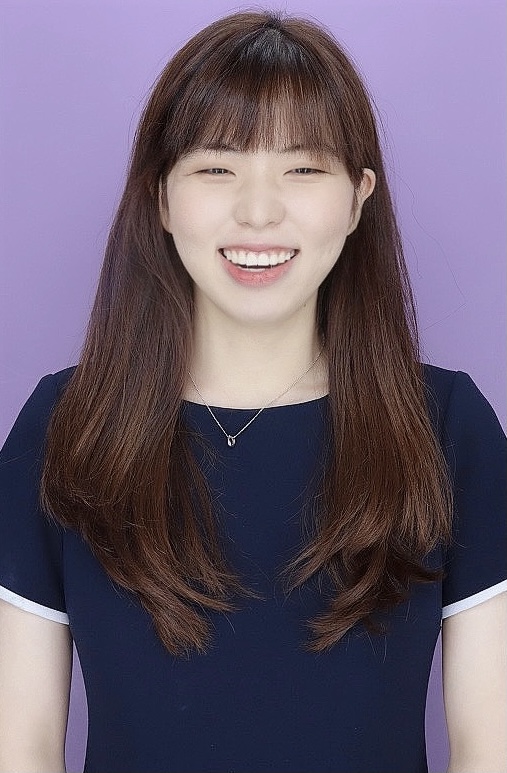
Cheeun Hong
Ph.D. Candidate
Department of Electrical and Computer Engineering, Seoul National University
Recently, many problems have been solved with deep learning using massive computational sources, often referred to as red AI. On the other hand, I am interested in green deep learning that considers energy usage and carbon emissions during model training and inference. Among the various compression technologies to obtain lightweight models, my previous works are mainly focused on efficient inference approaches such as network quantization and pruning. Specifically, several projects are about test-time adaptation of computational resources based on the sensitivity of the input image to compression (i.e., the less sensitive the input is, the fewer computational resources that are allocated). Although my latest projects are on compressing models for low-level image restoration problems, my research goal is to compress any deep learning model with massive computations.
Recent News
Feb 27, 2024 |
Our paper, AdaBM got accepted to CVPR 2024!
|
---|---|
Jul 25, 2023 |
Our paper, ODM is available on ArXiv.
|
Selected Publications
-
In reviewDifficulty, Plausibility, and Diversity: Dynamic Data-Free QuantizationIn Submitted for Review (In review), 2023
Education
Mar 2020 |
Seoul National University, South Korea Integrated Ph.D. in Electrical and Computer Engineering Advisor: Prof. Kyoung Mu Lee |
|
---|---|---|
Mar 2015 | ~Feb 2020 |
Seoul National University, South Korea B.S. in Electrical and Computer Engineering |