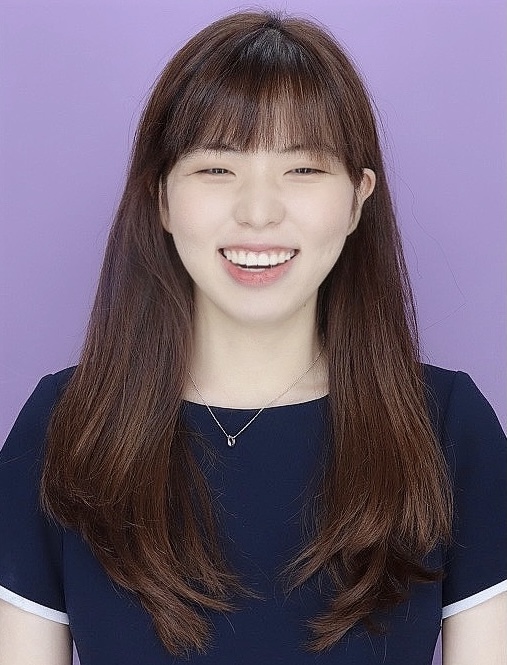
Cheeun Hong
Ph.D. Candidate @ Computer Vision Lab
Department of Electrical and Computer Engineering, Seoul National University
I am passionate about advancing efficient AI to optimize both model training and inference, with the ultimate goal of promoting sustainable AI. My research focuses on developing cutting-edge techniques like network quantization, pruning, and test-time adaptation, aimed at drastically reducing the computational costs while maintaining high performance. While much of my work has targeted efficiency improvements in low-level vision tasks like image restoration, my broader goal is to compress large-scale, computationally intensive models—including vision-language and generative models—to move closer to achieving on-device AI.
Keywords: Efficient AI, On-device AI
Recent News
Oct 2024 |
One first-co-authored paper on data-free quantization, DDPQ got accepted to WACV 2025.
|
---|---|
Jul 2024 |
One first-authored paper on quantization, ODM got accepted to ECCV 2024. See you in Milano!
|
Feb 2024 |
One first-authored paper on quantization, AdaBM got accepted to CVPR 2024. See you in Seattle!
|
Selected Publications
-
WACVDifficulty, Plausibility, and Diversity: Dynamic Data-Free QuantizationIn Winter Conference on Applications of Computer Vision (WACV), 2025
-
IEEE SPLCoLaNet: Adaptive Context and Latent Information Blending for Face Image InpaintingIn IEEE Signal Processing Letters (IEEE SPL), 2023
Education
Mar 2020 |
Seoul National University, South Korea Integrated Ph.D. in Electrical and Computer Engineering Advisor: Prof. Kyoung Mu Lee |
|
---|---|---|
Mar 2015 | ~Feb 2020 |
Seoul National University, South Korea B.S. in Electrical and Computer Engineering |